- SiMPL
- Posts
- SiMPL #012: Getting Lost to Learn
SiMPL #012: Getting Lost to Learn
Decisions, Detours, and Discovery
As we know, plans change just like we’ve talked about before. But here’s the fun part about learning: it’s a collection of life’s little assessments. We explored this concept in our previous newsletter, and here I am, living it out again.
Getting lost is honestly one of the best ways to learn your way around.
This week, I’ve been roaming around New York City. I’m a city walker—if a place is walkable and the weather’s decent, I’d rather walk than drive. And if public transportation is an option, that’s even better. Who needs a car?
This week, I’ve clocked in an average of 28,000 steps a day. Yes, that’s the real number. On the first day, I was on my feet for 18 hours—sounds wild, but I’m just that kind of tourist.
By Day 2, I started to feel at home, understanding how to navigate the streets, take the bus, and ride the subway. I knew how to catch the M15 to 1st Ave and E 50th St or hop on the E train to 53rd and Lexington. How did I get there? Google Maps helped, sure, but half the time, I didn’t trust walking around with my cell on my hand, and got myself lost! And that’s when those extra feelings kick in: you’re a little nervous, a bit insecure—“What if I end up in the wrong part of town? How much charge do I have on my phone? Wait, do I remember the address? And why is that guy playing the Dragon Ball theme song on a trumpet?”
Getting lost, for me, sparks a new level of awareness. I start paying closer attention to details, landmarks, street names—all the little things that help me get a sense of direction.
Coming from the urban chaos that is Panama City, NYC’s well-planned streets make it look easy. But even in the most organized city, learning your way still takes time and a few wrong turns. One day, a friend suggested I take the M1 or M2 bus to Central Park to reach the MET. I looked around and couldn’t find the bus stop, so I walked—beautiful day, no complaints! But after 20 blocks, you start to rethink your choices. On the way back, I found the bus stop right away, hopped on, but after sitting in brutal traffic, I got off and walked again.
But it all made sense because I knew my objective. Going to the MET that morning, I had time to spare, so I walked. Part of my plan was to stroll around Central Park after the museum, but hey, I ended up doing it on my way there instead.
In last week’s newsletter, we talked about decision-making—how humans make around 35,000 decisions a day. While in New York, those choices kicked into high gear. Where was I going? What time did the museum open? How long would it take to get there? Did my feet hurt? Was I hungry? Thirsty? In danger? These are all high-level, or “macro,” decisions.
Then there are the micro decisions—the ones you barely notice. Where do I step? Do I avoid the trash or steer clear of someone who seems a bit off? And, yes, sometimes I’d spot some good food, or a pretty girl passing by (I mean, we’re allowed to look, right? Haha, maybe that’ll come back to bite me on Sunday morning). Other micro choices: am I walking too fast or too slow? Should I check my watch, look at my phone? Did I forget my keys?
These aren’t one-off choices; they keep popping up throughout the day, all part of our everyday life journey.
All those little decisions we make every day are based on countless assessments—often requiring more data than we realize. A small choice needs at least three to five data points, so if you multiply that by our daily decisions, we’re looking at around 105,000 pieces of data! And we handle all that in a split second.
Do you consciously think through those data points when deciding whether to avoid stepping in, let’s say, dog poo? I hope it’s from a dog, now that I think about it. If you do consciously process it every time, shoot me an email; I’d love to hear your thought process.
For most of us, the aversion to stepping in something unpleasant feels automatic. We know it’s gross, but why? It might be that we were taught as kids to avoid messes. And maybe, way back, it had a different connotation. Imagine medieval streets full of horse manure—stepping in it was normal unless you were well-off enough to afford a ride above the muck. Monty Python’s “he must be a king because he’s not covered in mud” comes to mind!
In school, we learned the basics of computers: Input, Process, Output. That simple cycle has helped me understand life, too, and it’s probably why I gravitated toward process improvement projects. But even if we get the same input, our decisions differ wildly because our internal “process” varies for each of us.
Here’s a classic example: rats. We often have an instinctive disgust for them because they’re tied to one of history’s most infamous pandemics—the Black Death. If you’ve read The Plague by Camus, you’ll remember how it starts with rats swarming a city, foreshadowing an outbreak. (Spoiler: that’s our book recommendation this week.)
Now, think about how our decisions change just by tweaking the input. Imagine you’re walking through a park, and a creature runs up to greet you. If it’s a squirrel, you might smile; if it’s a rat, your response could be very different. Just reading these options shifts the situation entirely.
And let’s raise the stakes a bit: option c) a frog, or option d) a snake? The context changes instantly.
Picture this twist: You’re walking through the park, and a dog approaches you. Seems nice, right? But what if it’s a massive pit bull? Now you’re assessing everything in a flash—its body language, your comfort level, your distance.
If it’s Ozzy (my dog), you shouldn’t be worried. But how would you know that?

Ozzy!
So, we make decisions shaped by a mix of emotions, experiences, and biases, with so many complex layers that predicting them is incredibly challenging. Imagine trying to chart every little twist and turn of your thought process; you’d need a roadmap filled with tiny pathways.
Ever tried winning the lottery? It’s a bit like predicting human decisions—full of randomness and nearly impossible to crack. My late grandma had this “skill” where she would dream the winning numbers… but only after the draw. Suspicious, right? Some people find numbers in dreams, patterns in butterfly wings, even in astrology.
The reality is that the lottery is just a randomized set of numbers with so few “experiences” (draws) in history that it’s practically unpredictable. In Panama, we have a 4-number lottery drawn twice weekly, plus a special draw every quarter. The max payout is around $4,000 per ticket, and despite the slim odds, it’s practically a national pastime here.
If you put this through a machine, it would never “decide” to buy a ticket; the odds just aren’t worth it. Not even a “lucky” dream would sway the algorithm.
How do I know? Well, I’ve toyed around with predictive algorithms. I even built a Python script that pulled in historical lottery draws, transformed them into trends, and ran the numbers to see the best chances for repeats. The result? I predicted the last two digits once, which might happen again in another six months to a year. Not exactly a breakthrough.
Here’s the thing: machines also make decisions based on algorithms, just like we do, but with one crucial difference. Our “algorithms” are enhanced (or sometimes clouded) by all those factors—emotions, memories, biases. Machines don’t have those, and that’s where AI could theoretically step in to make cold, unbiased decisions.
Could this be the future? A world where AI helps us make the hard, sentiment-free decisions? It sounds promising, and I think it could lead us into a new era. Let’s see how it unfolds.
Why have organizations become so structured and standardized? It all goes back to the need for consistency in decision-making. From the earliest days of armies and mason guilds, leaders have relied on standardized processes to achieve predictable outcomes. Think about the pyramids—massive feats of engineering built 4,000 years ago—or the Roman Empire’s sprawling campaigns across continents. You couldn’t have a guy in the 4th regiment improvising directions in the woods while waiting for the cavalry; standards and protocols were essential.
Even in the face of the unexpected, like young Julius Caesar’s first encounter with those fearless Britons on the beach, there was a need for consistent, coordinated actions. Without standards, chaos would replace order, and the machine would fall apart.

Adventures of Asterix and Obelix
Later, as global empires grew—like the English East India Company—they needed to reduce individual thought in decision-making to ensure seamless operations. They couldn’t rely on feedback from across oceans; letters could take months to arrive, and conditions would have changed by then. So, they trained their ranks with clear processes, ensuring that any given input would lead to the same predictable output, no matter who handled it.
As time went on and education became widespread, and the Industrial Revolution sparked efficiency as a priority, the standardization of processes became essential. Workers were trained in specific tasks, repeating them hour after hour to achieve perfection through efficiency.
This is also why forms exist. Those forms you fill out at the DMV or in customs? They’re filled with the exact pieces of information needed to reach a standardized decision quickly. By filtering the data through specific questions, organizations can remove most of the guesswork.
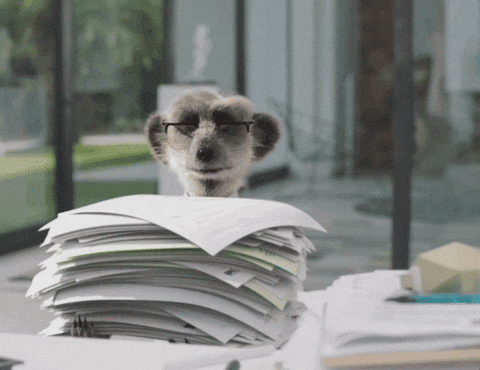
Gif by comparethemarket on Giphy
But here’s the thing—how many of those forms are necessary now? If all that data is stored somewhere, already digitized, how many forms, mid-management positions, and bureaucratic structures do we really need to make decisions? The systems we rely on are designed to limit variance, but with data access improving, there’s an opportunity to rethink whether we need all that paperwork and oversight.
Imagine what would happen if the decision-making process could be streamlined, pulling data automatically without the need for endless layers of approvals.
In a previous newsletter, we discussed how Ajay Agrawal, Avi Goldfarb, and Joshua Gans in their Book: Power and Prediction: The Disruptive Economics of Artificial Intelligence, pointed out that AI models were tested with the same initial data available to decision-makers at the onset of COVID. The aim was to see if AI might have responded differently than humans, who relied heavily on historical precedents, like the 1918 Spanish flu. Back then, certain towns had used masks and social distancing to curb the spread, creating a playbook for us.
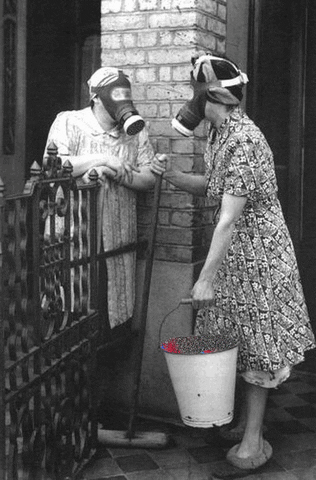
Gif by alinasanchez on Giphy
But as we gathered new data during COVID, that information had to filter through layers of politics and bureaucracy. By the time decisions were made, the data was often outdated. Protocols required slow-moving approvals and adaptations that weren’t built for the speed needed during a pandemic of this scale.
At my job, I served on the Health Committee, adjusting processes to face the reality of the pandemic. We prepped for everything: remote work, electronic signatures, data loss prevention, ergonomics, and more. In theory, we were ready, but adjusting to this reality took time and highlighted gaps in our continuity plans, which had mainly considered natural disasters, power outages, or political disruptions—not pandemics of this magnitude.
As referenced in Power and Prediction case study, AI cut straight through these procedural layers, discarding unnecessary steps to use the latest data immediately. AI models indicated that, rather than shutting down entirely, letting the virus spread under monitored conditions might have built immunity more effectively and minimized certain lockdown measures. But we acted out of caution, sticking with familiar strategies instead of exploring new possibilities.
This glimpse into AI’s potential highlights that, while models aren’t yet refined for every daily decision, they hold promise. Right now, we’re just past the hype phase in the adoption curve. This period could give us the breathing room to develop real, practical use cases, moving beyond the hype and addressing fundamental issues like energy use, sustainable materials, and better data modeling. These improvements are essential before AI can truly take on complex, nuanced decision-making at scale.
But let’s explore a current AI use case: self-driving cars.
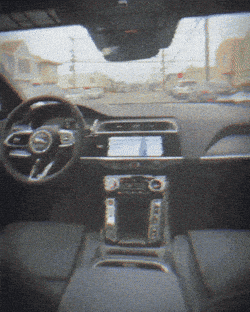
Gif by yevbel on Giphy
Last year in Phoenix, I had the chance to ride in one of Waymo’s self-driving Jaguars. Sitting there, I remember telling my wife, “This thing drives better than me.” It even paused to make sure all passengers had their seatbelts on before it moved. Impressive, right? The car handled everything—speeding up, slowing down, spotting risks, and braking. It even respected traffic lights and maintained a courteous distance from other cars. But should it be that impressive?

Driving rules are straightforward, and they don’t vary too wildly from country to country. There’s always a manual, and whether you’re driving in New York or on the left side in England, the fundamentals are similar. What complicates things are the unexpected moments—a dog darting across the street, a broken stoplight, a reckless driver. And that’s not even considering each driver’s vision, reflexes, or vehicle condition.
So, how does the Waymo car handle it? It’s outfitted with a ton of sensors feeding data into a machine-learning algorithm. It learned by observing thousands of casual drivers maneuver around cities, making mistakes, taking risks. A set of trainers would review these driving behaviors, flagging what to avoid and reinforcing positive driving actions. This feedback loop trained the algorithm over countless hours.
Machine learning, though, isn’t magic—it’s experience-based. For my dissertation back in 2008, I used a method called a Genetic Algorithm, designed to correct errors generationally, by testing and refining solutions over iterations. Each generation of the algorithm “learns” by mutation and improvement, gradually getting better at solving the problem. We used it for error correction on hard drive CPUs, but the principle is similar in machine learning. Waymo’s algorithm takes those learning principles, evolving its “driving” through feedback and testing thousands of situations until it adapts and improves.
What’s even more interesting is that all this data doesn’t sit in isolation. Waymo centralizes it, pulling in information from every car to refine the collective driving experience. It’s like if every driver could tap into a shared pool of road wisdom—a super-powered, real-time Reddit of driving knowledge. But, honestly, a life that’s that pre-calculated doesn’t sound appealing.
I love the spontaneity of making my own decisions—the detours, mistakes, and lessons learned along the way. It’s a lot like making espresso. Last year, I bought a 1969 La Pavoni, a vintage lever machine with no shortcuts and plenty of room for error. In the beginning, I burned through nearly 20 pounds of coffee beans, ruining shot after shot. But through cycles of trial and error, frustration, small wins, and constant tweaking, I eventually pulled a shot I could be proud of. Every misstep led to that first really decent espresso—and it made the whole journey worthwhile.
There are machines that could make a flawless espresso at the touch of a button or GPS that could prevent me from ever getting lost. But I prefer the surprises—getting lost in a new city, discarding bitter shots for the pursuit of perfection. Those unexpected moments remind me that life’s beauty isn’t just in efficiency; it’s in the mess of figuring things out, each decision leading to experiences I wouldn’t trade.
So, call me old-school—I love figuring things out on my own, getting lost in the process, embracing the unexpected twists, and learning from my mistakes. There are just some things I’d rather keep doing myself.
But… I’ll admit, I still used AI to help me with the grammar on this piece.
Book Recommendation: The Plague by Albert Camus
This week, let’s dive into a classic that feels eerily relevant: The Plague by Albert Camus. I picked it up again during the pandemic—because, you know, why not add a fictional epidemic to the real one? Halfway through, I thought, “Great choice, self,” as the book mirrored our chaos a bit too well. Camus masterfully captures the confusion, political madness, and the spectrum of human reactions during a crisis. It’s like he had a front-row seat to 2020.

Our household became its own mini-version of Camus’s quarantined town. My grandma, in her 80s and unable to walk, usually had a daytime caretaker. But once COVID hit, her caretaker, understandably scared, decided to stay home. So, we scrambled to adjust. My brother-in-law was starting his career, juggling a call center job while hunting for the perfect gig. My wife had the unenviable task of laying off staff remotely while her boss was stuck in Mexico. And I was working from home, trying to keep it all together. Our living room transformed from a relaxation zone to a stress hub, much like the town square in Camus’s novel.
In The Plague, Dr. Rieux observes, “There have been as many plagues as wars in history; yet always plagues and wars take people equally by surprise.” Sound familiar? Despite history’s lessons, we often find ourselves unprepared when disaster strikes. The characters in the book make decisions fueled by empathy, fear, selfishness, and hope—emotions we’ve all wrestled with recently.
Camus’s portrayal of a community facing an invisible enemy resonates with our experiences. It’s a reminder that while we can’t predict every twist life throws at us, our choices define how we navigate the chaos. If you’re up for a read that offers both reflection and a touch of “I’ve been there,” The Plague is worth your time. Plus, it’s always fun to see how much—or how little—things have changed since 1947.
SiMPL’s Weekly World Wrap-Up (Nov17th /24)
Golden Arches Go on Damage Control McDonald’s is dropping $100 million to bounce back after an E. coli outbreak linked to slivered onions on its Quarter Pounders. The budget splits into $35 million for marketing (hello, $1 McNugget deals!) and $65 million to help franchisees in affected areas. With over 100 people sickened and lawsuits piling up, McDonald’s is pulling out all the stops to restore trust and taste buds. Want the full scoop? Check out the article here. | Amazon’s Smart Glasses: Barking Up a New Tree Amazon wants to make ’90s delivery nightmares—startled by bloodthirsty Rottweilers—a thing of the past. Enter Echo Frames smart glasses for drivers, designed to guide them through buildings and dodge big scary dogs. These specs offer turn-by-turn navigation and could shave off precious seconds on deliveries, adding up to greater efficiency. But there’s a snag (or three): • Battery life struggles to last an 8-hour shift. • Mapping out houses and surroundings could take years. • Drivers might resist wearing heavy, clunky glasses. With fewer than 10,000 Echo Frames sold to consumers, Amazon has some hurdles ahead—while Meta and Apple race toward their own smart glass futures. |
Spain’s Devastating Floods: A Grim Recovery Paiporta, a town on Valencia’s outskirts, is grappling with the aftermath of catastrophic flooding that claimed over 60 lives and left residents devastated. A sudden river surge, triggered by torrential rain upstream, swept through homes, vehicles, and streets, catching residents off guard. • Hundreds remain displaced, with no running water or electricity. • Rescuers continue to retrieve bodies from mud-filled homes and garages. • Survivors lament the lack of warnings and swift support. As Paiporta mourns its losses, questions arise about preparedness and the devastating power of natural disasters.Read The New York Times amazing story here. | Bluesky: The Cool Kid on the Block Tired of Musk’s X-periments? Enter Bluesky, the social platform gaining traction among ex-X users looking for an ad-free, friendlier vibe. • Bluesky, backed by Twitter’s original CEO Jack Dorsey, has grown to 15 million users. • It’s nostalgic for early Twitter days: chronological feeds, pinned posts, and meme-sharing galore. • Bluesky’s bigger goal? Interoperability—social networks that talk to each other like email. Is Bluesky the future of social media or just a nostalgic rebound? Dive in here. |